Below are some of the topics I have worked on since beginning my DPhil, in approximately anti-chronological order. A complete list of my publications can be found on Google Scholar.
Multi-Agent Risks from Advanced AI
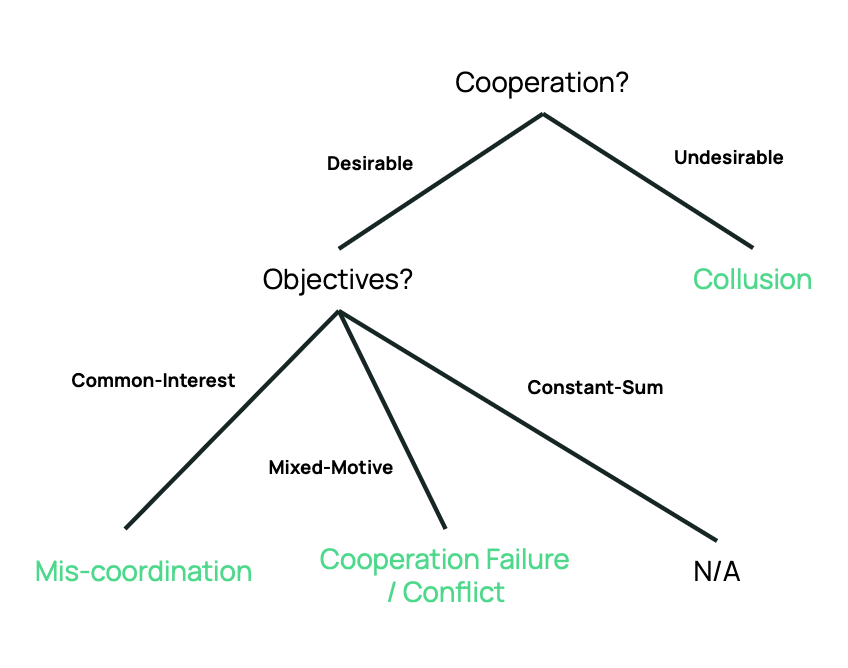
It is likely that we will soon see advanced AI systems increasingly coming into contact both with each other and with humans, sometimes in high-stakes settings such as financial markets or military domains. These new multi-agent systems present qualitatively different kinds of risks, which thus far have been systematically underappreciated and understudied.
Agents Verifying Agents
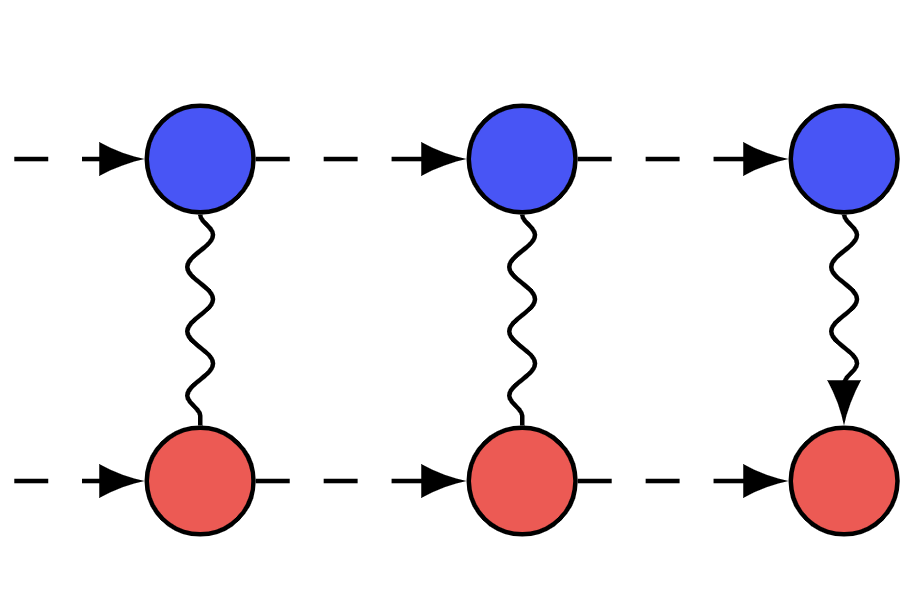
As our AI agents become ever more sophisticated and ubiquitous, it is critical that we are able to provide guarantees that they will act safely. Unfortunately, most classical verification methods for doing so do not scale to the vast AI systems being built today. One alternative is to reframe the verification problem by using AI agents to verify each other.
Preventing AI Collusion

While it is often the case that we want AI systems to cooperate with each other, in some settings (such as markets) cooperation is undesirable. This raises the question of how we might define, detect, and mitigate against the risk of AI collusion. This is especially challenging in settings where AI systems might be able to collude secretly via steganography.
AI for Institutions
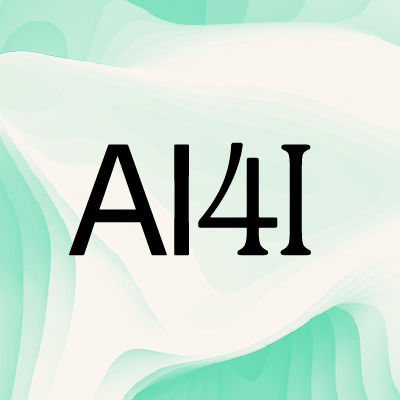
AI and other technologies are rapidly evolving, outpacing our institutions’ abilities to address risks and opportunities. Could AI itself be used to imagine and build new institutions, so we can better collaborate, govern, and live together? The first AI for Institutions workshop was organised in 2023 by the Collective Intelligence Project and the Cooperative AI Foundation.
Evaluating Cooperative Intelligence
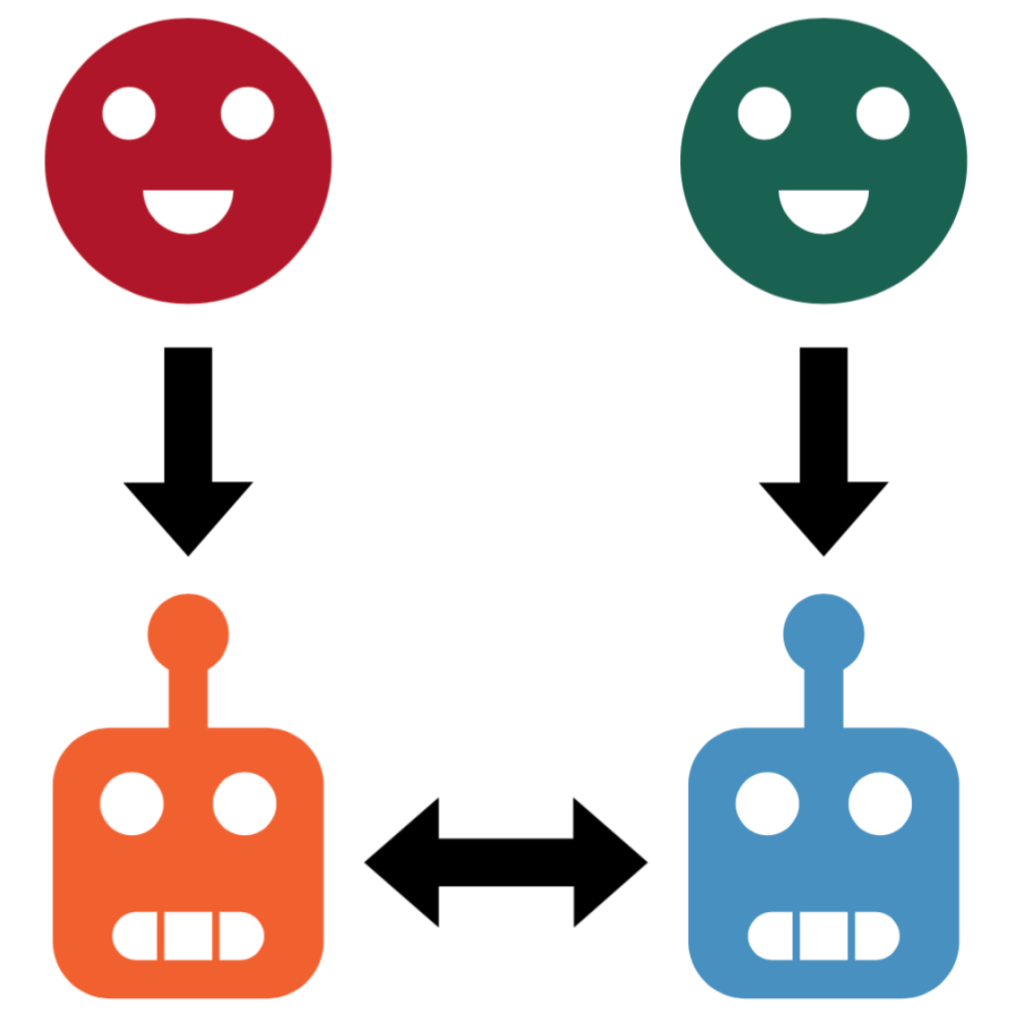
How can we tell when one agent is being more cooperative than another? More specifically, how might we measure both the cooperative capabilities and dispositions of AI systems? Answering this question in practice requires both theoretical analysis and empirical benchmarks that can be applied to state-of-the-art systems such as large language models.
Causal Models of Games
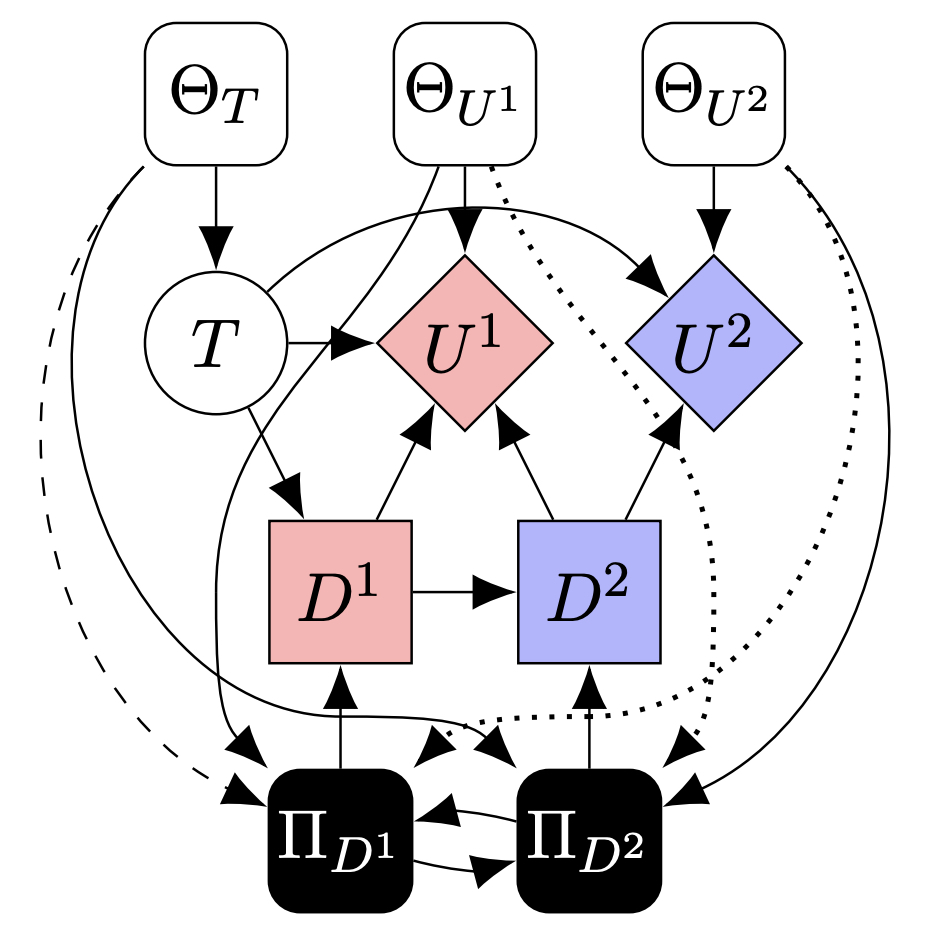
Causal games unify two broad topics in AI – causality and game theory – by generalising multi-agent influence diagrams to higher levels of Pearl’s ‘causal hierarchy’. Together with additional game-theoretic developments, these models can be used to reason about a range of important (and implicitly causal) concepts, such as intention, agency, harm, and blame.
Rational Verification
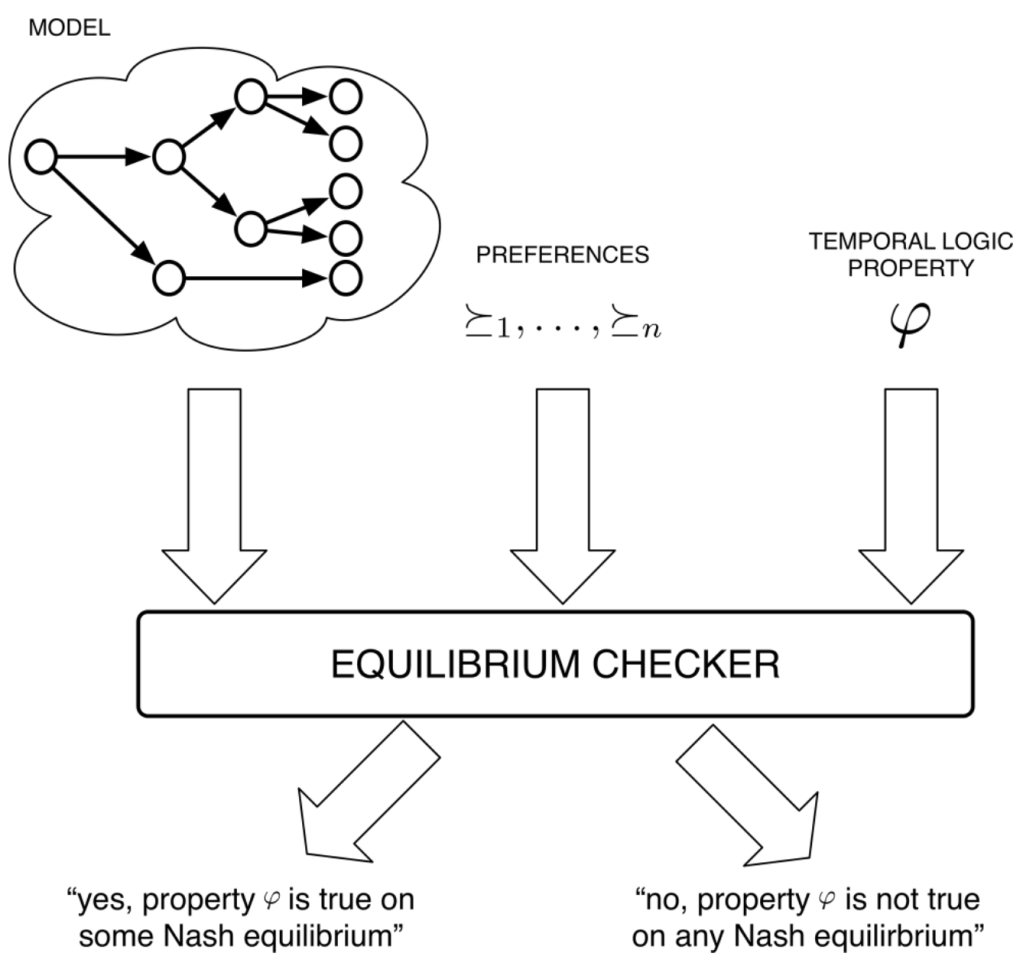
In standard model-checking, we take a model of a given system and compare it against a specification that we want that system to satisfy. In (potentially probabilistic) systems that contain multiple strategic agents, we instead need to check that the specification holds under the game-theoretic equilibria of the system, in a process known as rational verification.
Non-Markovian Rewards in Reinforcement Learning
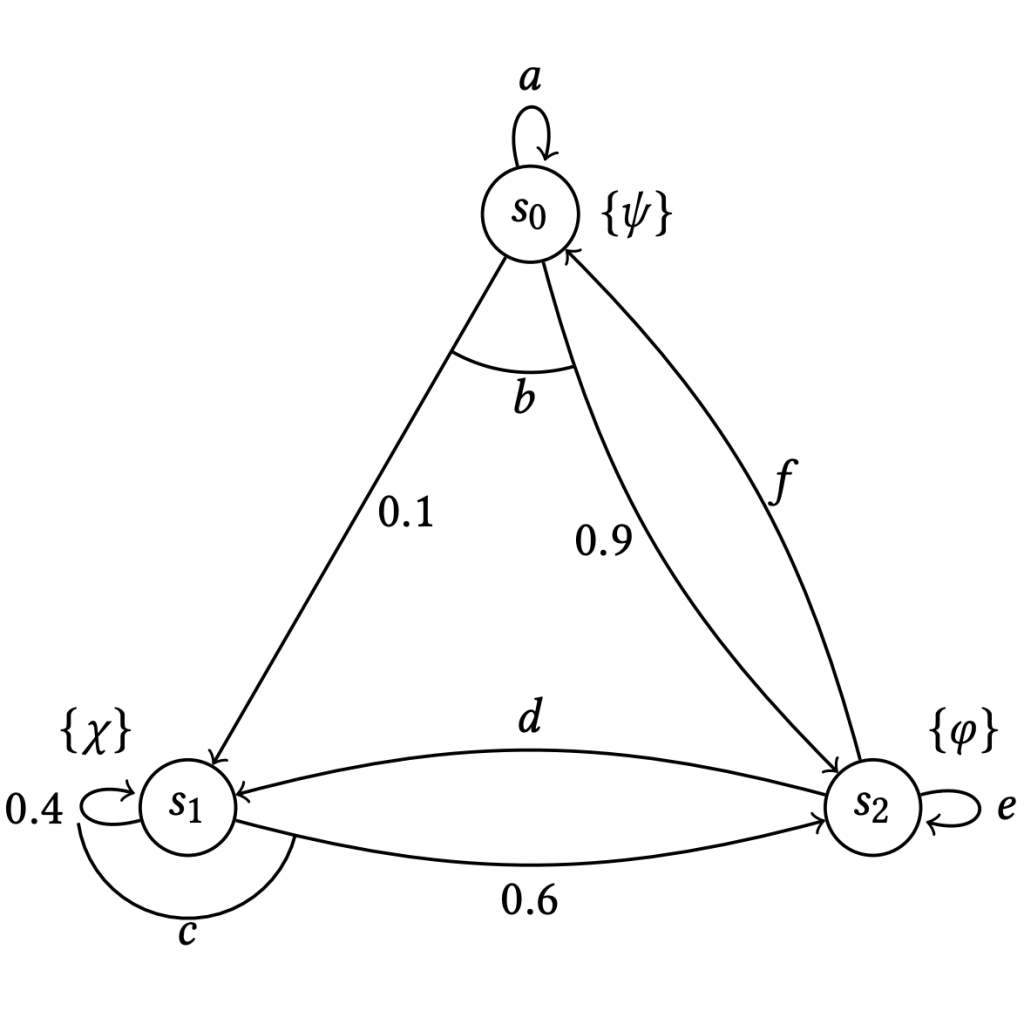
One of the fundamental difficulties in reinforcement learning is correctly specifying the agent’s reward function so as to incentivise desirable behaviour. This difficulty can be partially overcome by using non-Markovian reward signals, such as temporal logic formulae, or a lexicographic sequence of scalar reward functions.